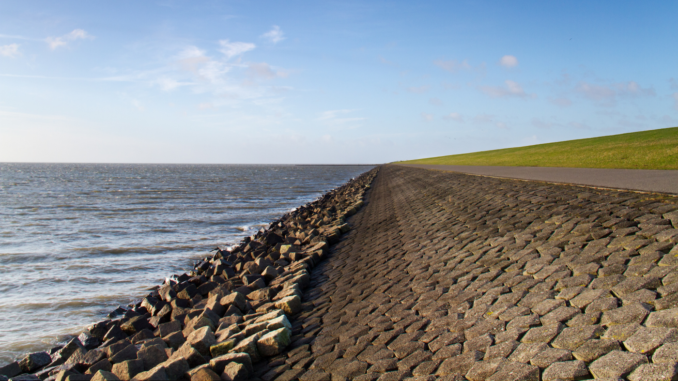
The article “Sea Level Rise Learning Scenarios for Adaptive Decision-Making Based on IPCC AR6” was published in Earth’s Future.
In many cases of climate change adaptation, substantial investments in infrastructure (e.g., dikes) are necessary. However, the uncertainty surrounding future projections, such as sea level rise, poses significant challenges. Decision scientists tackle these issues by employing flexible and staged decision-making methods. For instance, a coastal decision-maker might opt to construct a dike with a broader foundation initially and, if required, increase its height in the future. This approach allows decision-makers to gain insights by monitoring future sea level rise trends to determine if higher dike protection levels are warranted.
To assess whether it is economically advantageous to defer infrastructure investments in favor of learning from future observations, which could justify additional expenses for flexible infrastructure, it is essential to establish learning scenarios. Learning scenarios involve projecting critical variables from the current perspective and from various future timeframes. For instance, sea level rise learning scenarios encompass projections of sea level rise from 2050 onward, contingent on the amount of sea level rise observed up to 2050.
This paper aims to elucidate coastal decision-making employing a learning scenario through a straightforward example. Additionally, it introduces a novel method for generating learning scenarios and applies this method to create sea level rise learning scenarios.
Key points:
- We develop sea-level rise learning scenarios based on IPCC AR6 using a novel method termed direct fit.
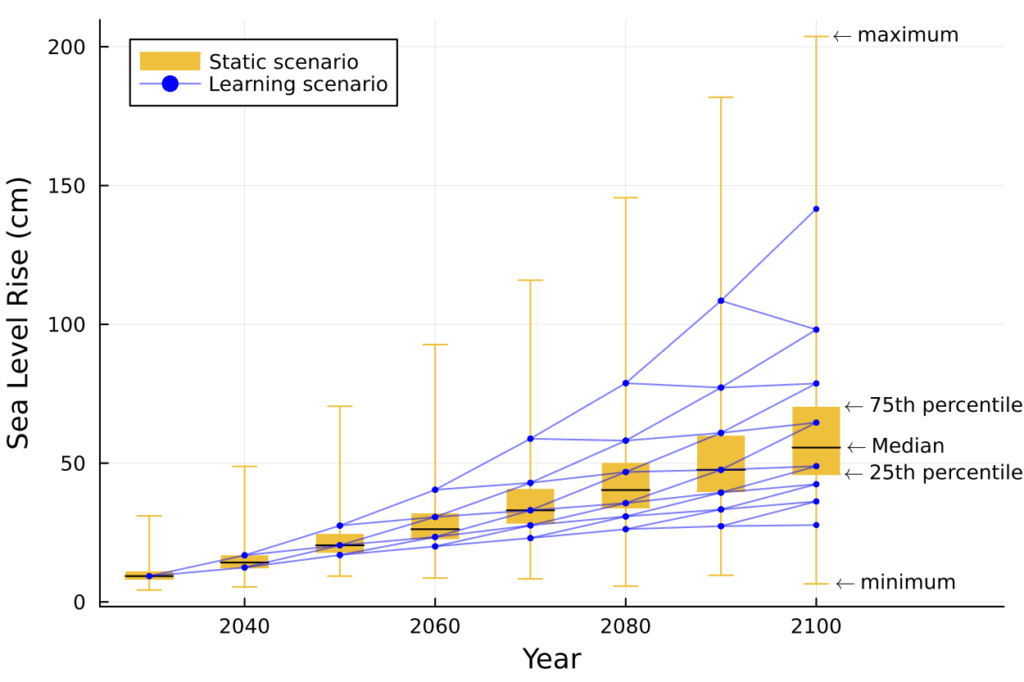
- Learning scenarios provide information on future variable values seen not only from today, but also from future moments in time. The information seen from a future moment in time is an updated estimation based on learning, e.g. observations, until that future moment in time.
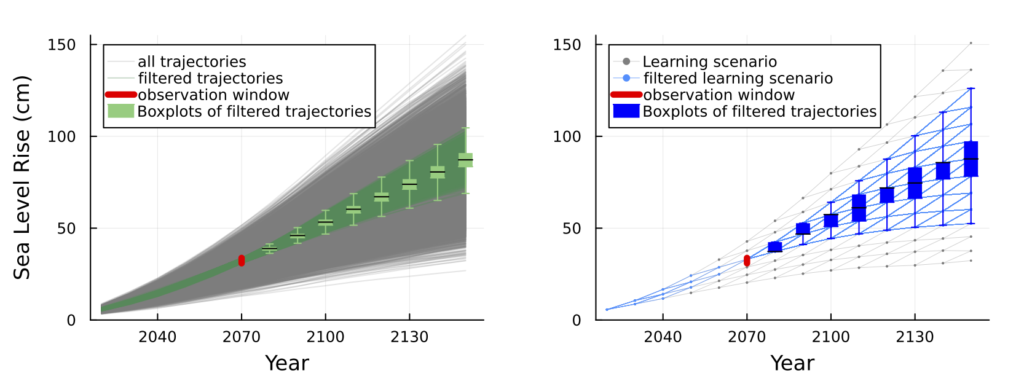
- We show how climate learning scenarios can be applied for improving and justifying investments in flexible long-lasting infrastructure. This figure visualises how sea level rise learning scenarios extend adaptation pathway analysis.
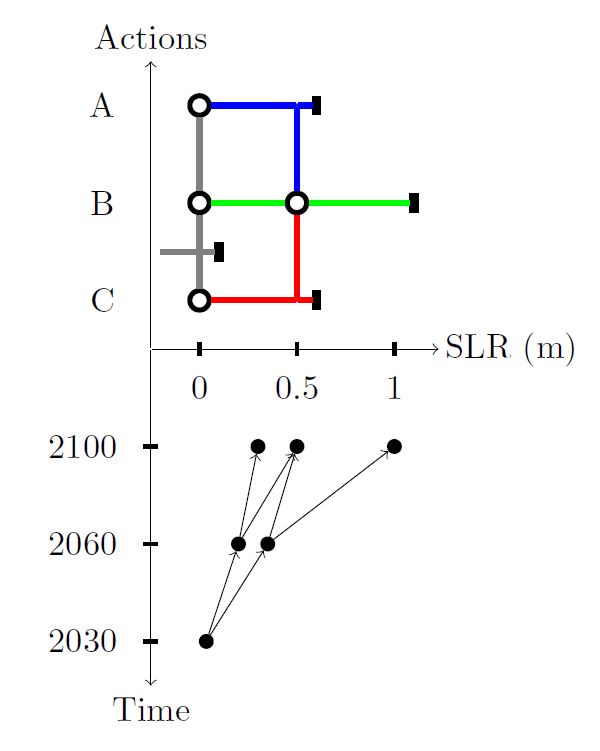
Leave a Reply