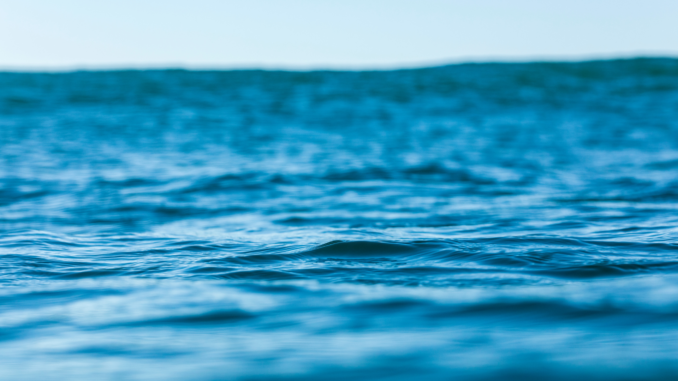
The article “Improving Statistical Projections of Ocean Dynamic Sea-level Change Using Pattern Recognition Techniques” has been published in Ocean Science.
Climate change will alter heat, freshwater fluxes and ocean circulation, driving local changes in sea level. This sea-level change component is known as ocean dynamic sea level (DSL), and it is usually projected using computationally demanding Global Climate Models (GCMs).
Statistical models based on GCM simulations can be a more efficient alternative for projecting DSL but may contain significant errors. Here, we partly remove those errors (driven by internal climate variability) by using pattern recognition techniques applied to a large ensemble.
This enables us to:
- reduce statistical errors more efficiently (i.e., using less realizations) than conventional approaches, such as ensemble averaging. We found filtering 2 ensemble members yields the same performance as averaging 12 from the 100-member MPI-GE model.
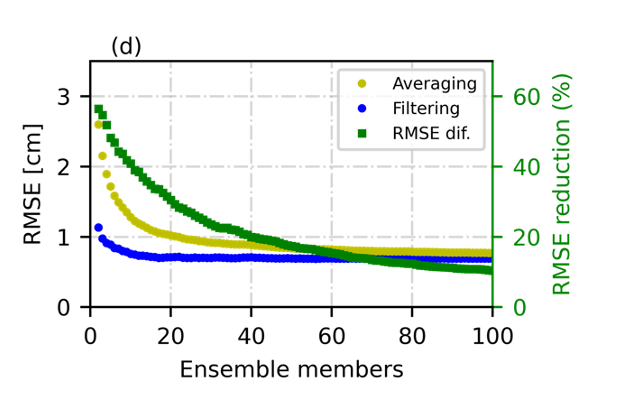
- improve statistical projections in single-realization modelling experiments, especially in places subject to high internal climate variability (e.g., western boundary currents).
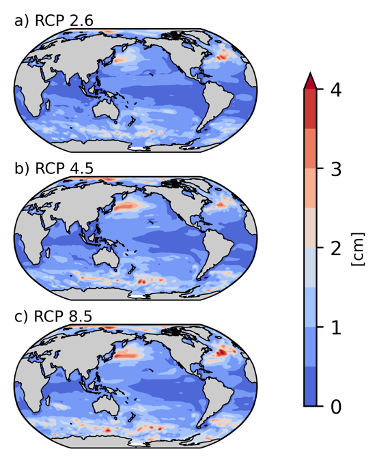
Leave a Reply