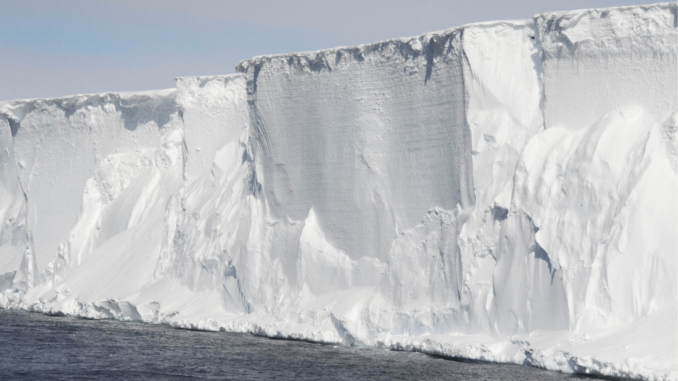
The article “Emulating Present and Future Simulations of Melt Rates at the Base of Antarctic Ice Shelves With Neural Networks” was published.
It presents a new method based on artificial intelligence that could help accelerate projections of polar ice melt and future sea level rise.
The problem
Melt rates at the base of Antarctic ice shelves are needed to drive projections of the Antarctic ice sheet mass loss. However current basal melt parameterizations struggle to link open ocean properties to ice-shelf basal melt rates for the range of current sub-shelf cavity geometries around Antarctica.
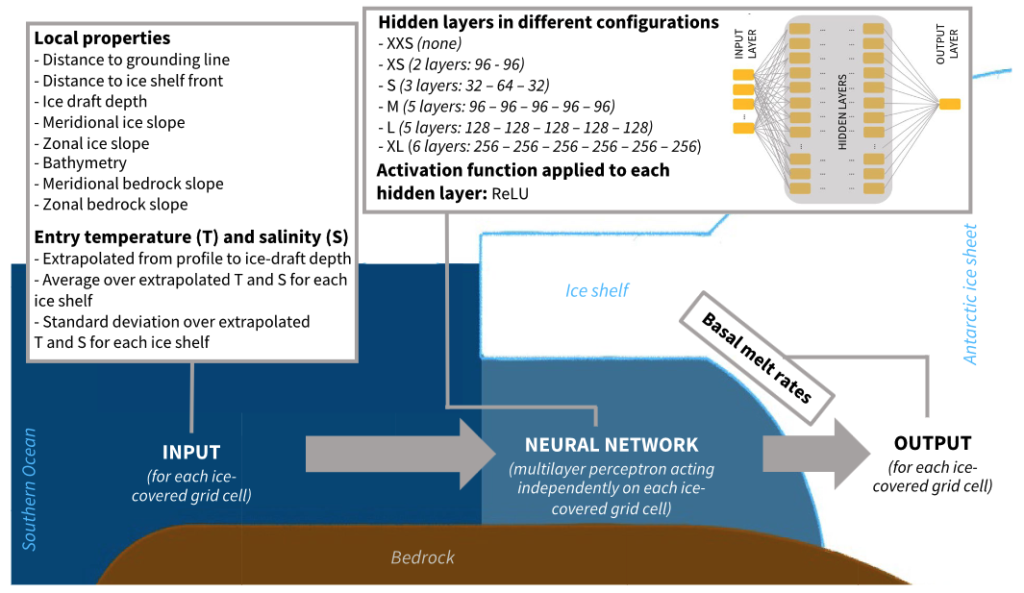
What we do…
We present a proof of concept exploring the potential of simple deep learning techniques to parameterize basal melt. We train a simple feedforward neural network to emulate the behavior of circum-Antarctic cavity-resolving NEMO ocean simulations.
We find that comparably small neural networks applied on the grid-cell level emulate well basal melt rates in near-present conditions, generalising better to time steps unseen during training than to geometries unseen during training.
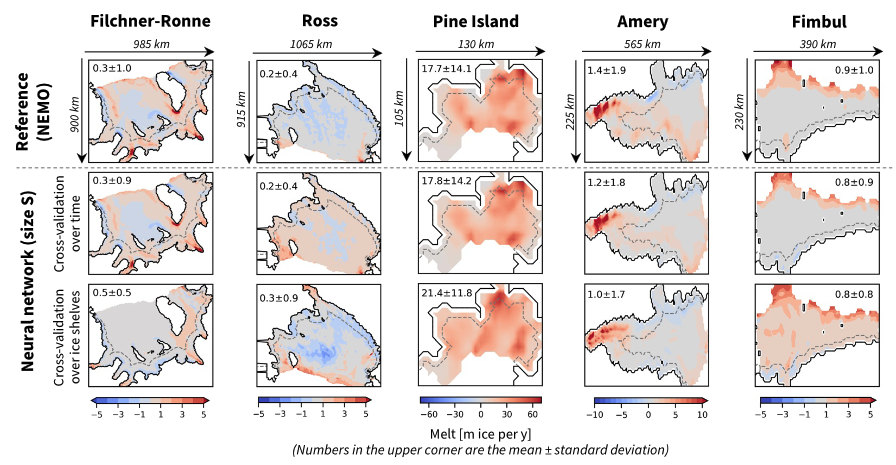
We find that, for much warmer conditions, both neural networks and traditional parameterisations struggle. While this shows that such a neural network is at least as suitable for century-scale Antarctic ice-sheet projections as traditional parameterizations, it also highlights that tuning any parameterization on present-like conditions can introduce biases and should be used with care.
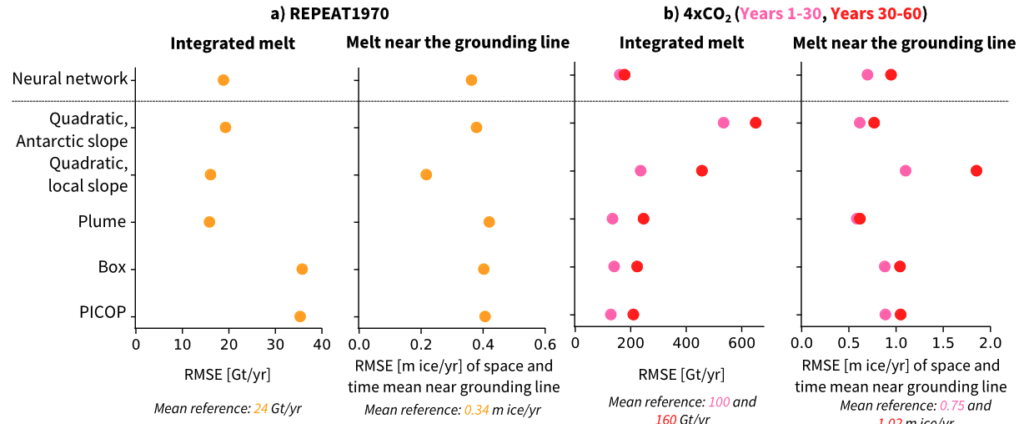
In summary, this proof of concept is promising and provides a basis for further development of a deep learning basal melt parameterisation.
To go further…
Read the editor’s highlight in Eos: Deep Learning Tackles Deep Uncertainty
Leave a Reply